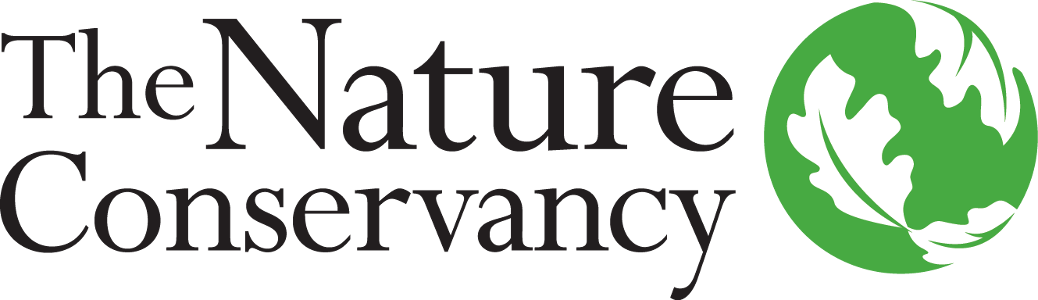
Diego J. Lizcano, Ph.D.
Unillanos, Villavicencio
Simplificación de un sistema real.
Permite entender como interactuan o se afectan los parametros si variamos algo.
Como el tamanio de la muestra afecta la distribución de la media?. Usaremos el set de datos iris de R y la columna largo del petalo.
str(iris)
iter <- 1000 # Numero de iteraciones
n <- 1 # numero de datos que muestreo. Variar hasta 150
means <- rep(NA, iter) # aca almaceno las medias de cada iteracion
for (i in 1:iter){
d <- sample(iris$Petal.Length, n) # sample toma n (1) datos
means[i] <- mean(d) # saca la media y guarda en means
}
hist(means)
abline(v=mean(iris$Petal.Length), lty=2, lwd=3, col="blue")
\[ \begin{aligned} y = \alpha + \beta x + \epsilon \end{aligned} \]
donde \(\alpha\) y \(\beta\) son parametros del modelo y \(\epsilon\) el error del modelo.
\(\alpha\) corresponde al intercepto
\(\beta\) corresponde al coeficiente de x (pendiente).
cuando \(\beta\) = 0, no hay relación significativa entre las variables x y y.
Usaremos el set de datos iris de R.
str(iris)
model1 <- lm(Sepal.Length~Petal.Length, data=iris)
summary(model1)
library(ggplot2)
ggplot(model1, aes(x=Petal.Length, y=Sepal.Length)) + geom_point() +
geom_smooth(method = "lm") # try geom_smooth()
newdato <- data.frame(Petal.Length=seq(4, 7, by=0.1))
predict(model1, newdata = newdato) # predice sepalo cuando petalo es de 4 a 7
Usaremos el set de datos iris de R. Use + para combinar efectos. : para interacciones A:B; y asterisco (*) para efectos e interacciones, ej A*B = A+B+A:B
str(iris)
model1a <- lm(Sepal.Length~Petal.Length * Petal.Width, data=iris)
summary(model1a)
library(lattice)
newdato<-expand.grid(list(Petal.Length = seq(4, 7, length.out=100),
Petal.Width=seq(1, 2.5, length.out=100)))
newdato$Sepal.Length<-predict(model1a, newdata = newdato) # predice sepalo con petalo de 4 a 7 y 1 a 2.5
levelplot(Sepal.Length~Petal.Length + Petal.Width, data=newdato,
xlab = "Petal.Length", ylab = "Petal.Width",
main = "Surface of Sepal.Length")
Usaremos el set de datos mtcars de R.
data(mtcars)
model2 <- glm(vs~mpg, data=mtcars, family = binomial(link = "logit"))
summary(model2)
library(ggplot2)
ggplot(mtcars, aes(x=mpg, y=vs)) + geom_point() +
geom_smooth(method = "glm", method.args = list(family = "binomial"))
newdato2 <- data.frame(mpg= seq(20, 30, by=0.5))
predict(model2, newdata = newdato2, type='response') # predice vs cuando mpg es de 20 a 30